Machine Vision Search is a prototype search system for identifying objects and actions in archival video. The software leverages image recognition algorithms to enable content-based search and metadata generation in video collections. The system uses Google images generated from a given search word to train a neural network. Once trained, the network can find other examples of the concepts that were tagged in larger film libraries.
The Machine Vision Search system was designed to explore automated tagging and categorization in educational institutions, libraries or anyone interested in sustained scholarship and preservation of film archives. It was created to lower the technological and expertise requirements needed to deploy machine learning in archives. This new search strategy could change how video content is discovered online.
MEP has partnered with the Visual Learning Group to research methods to learn models of the real-world from visual data, such as images, video, and motion sequences. Our collabortion leverages the framework of deep learning to address challenging problems at the boundary between computer vision and machine learning including image categorization, action recognition, depth estimation from single photo, as well as 3D reconstruction of human movement from monocular video.
MVS was made possible with the generous support of the Knight Foundation and in partnership with the Internet Archive, Visual Learning Group, and DALI Lab.
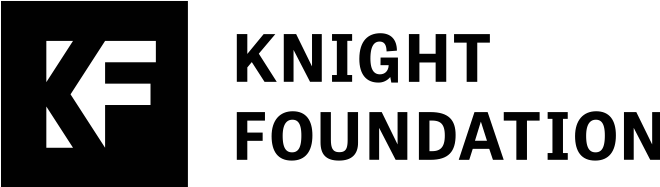
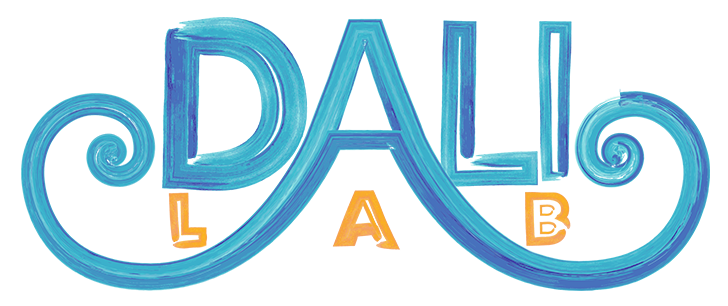
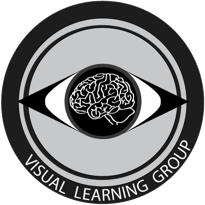
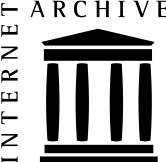